Trait Spotting
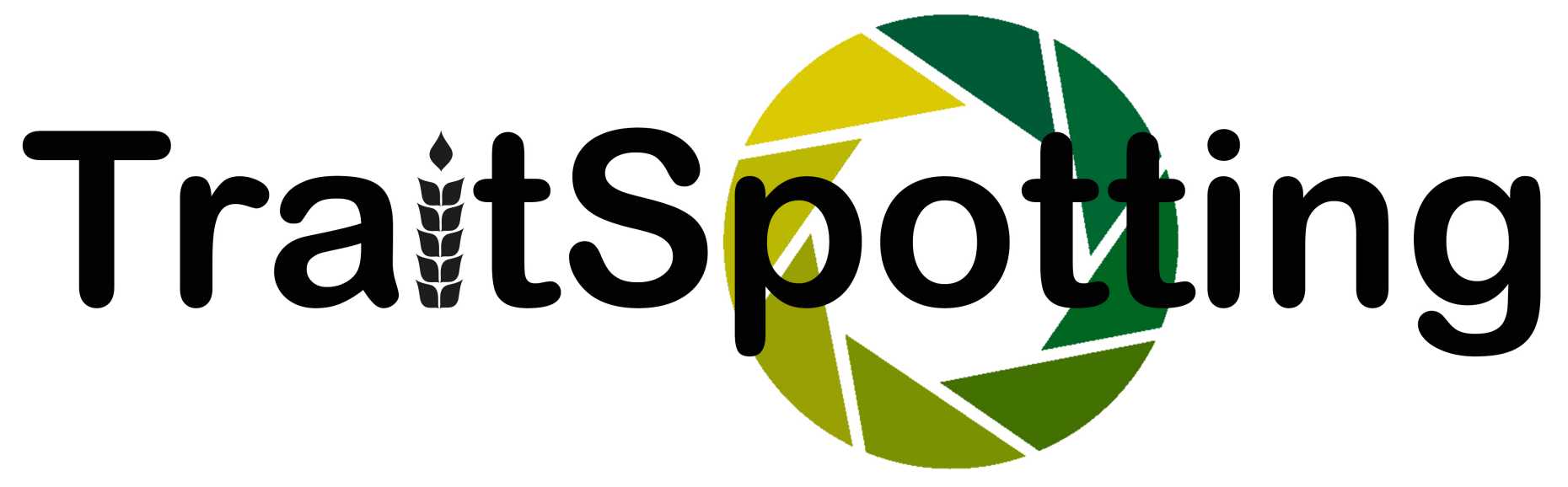
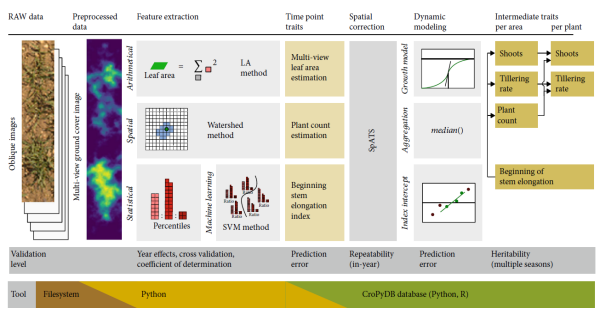
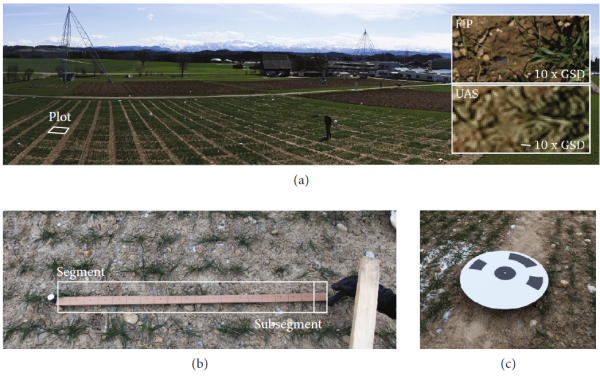
Crop yield has to be enhanced by 38 % within the coming decades to secure the expected global food demand in 2050 (external page Tester and Langridge 2010). Thus, more food needs to be produced using sustainable intensification strategies to avoid waste of finite resources and to stop conversion of new land to agriculture (external page Godfray and Garnett 2014). Breeding in combination with intense agricultural research will play an important role to achieve these aims by adapting crops to lower inputs of agrochemicals, integrate them into new crop rotations and keep pace with the emergence of new pests and diseases. In addition to the challenges of global climate change, breeders are faced with an increasing “shopping list” of new target traits that need to be taken into account when breeding for a sustainable intensification. As a consequence, breeders today have to invest considerably more time and effort to sustain yield increase than during the last century (external page Morris, Edmeades, and Pehu 2006) and costs of field phenotyping have become a major factor.
Within Traitspotting, ETH Zurich, Delley Seeds and Plants and GAMAYA teamed up to tackle these challenges by means of drone-based phenotyping.
The team developed phenotyping protocols to measure target traits in the visual, near-infrared and long-wave infrared part of the electromagnetic spectrum. Depending on the trait and sensor, different levels of technology readiness were achieved.
The most advanced methodology relates to high-resolution, multi-view visual imaging of the crop canopies. A workflow was established that includes flight planning (Roth, Hund, and Aasen 2018), photogrammetry and feature extraction, followed by spatial correction and dynamic modelling to extract intermediate traits (external page Roth et al. 2021). By using jointly collected ground truth data, we established and calibrated feature extraction workflows for different target traits. In wheat we stashed routines to quantify early canopy cover and tillering intensity (Roth et al. 2020). In soybean, the early canopy cover development, leaf area index and senescence behaviour are now routinely assessed.
The measurement of canopy temperature was brought to an intermediate level of readiness: A workflow was established (Perich et al. 2020) and an advanced feature extraction method using dynamic trends in temperature and senescence during early grain filling was developed (Anderegg et al. 2021). However, we observed confounding effects with traits, such as plant height. When taking these confounding traits into account, the remaining differences in canopy temperature showed a low heritability across years. Thus, further research is needed to evaluate the reason for this genotype-by-year interaction.
Hyperspectral imaging and specialized indices related to the dynamics of canopy senescence (Anderegg et al. 2020) or disease severity (Anderegg et al. 2019) hold great promise for routine breeding. As part of this project, GAMAYA was able to identify and remove limitations in the data capturing workflow of their hyperspectral imaging sensor. These findings in combination with the collect ground truth data will empower GAMAYA to develop and provide competitive commercial solutions to classify and quantify plant characteristics.
Aims
- Develop and release a tool-set that supports users in planing and performing successful UAV based remote sensing missions
- Establish a data processing pipeline to extract data from photogrammetric products and individual oblique images
- Develop methods to extract traits
Partners
- Crop Science group of ETH Zurich
- external page Delley Seeds and Plants
- external page Gamaya
Funding and support
Output