Dr. Lukas Roth
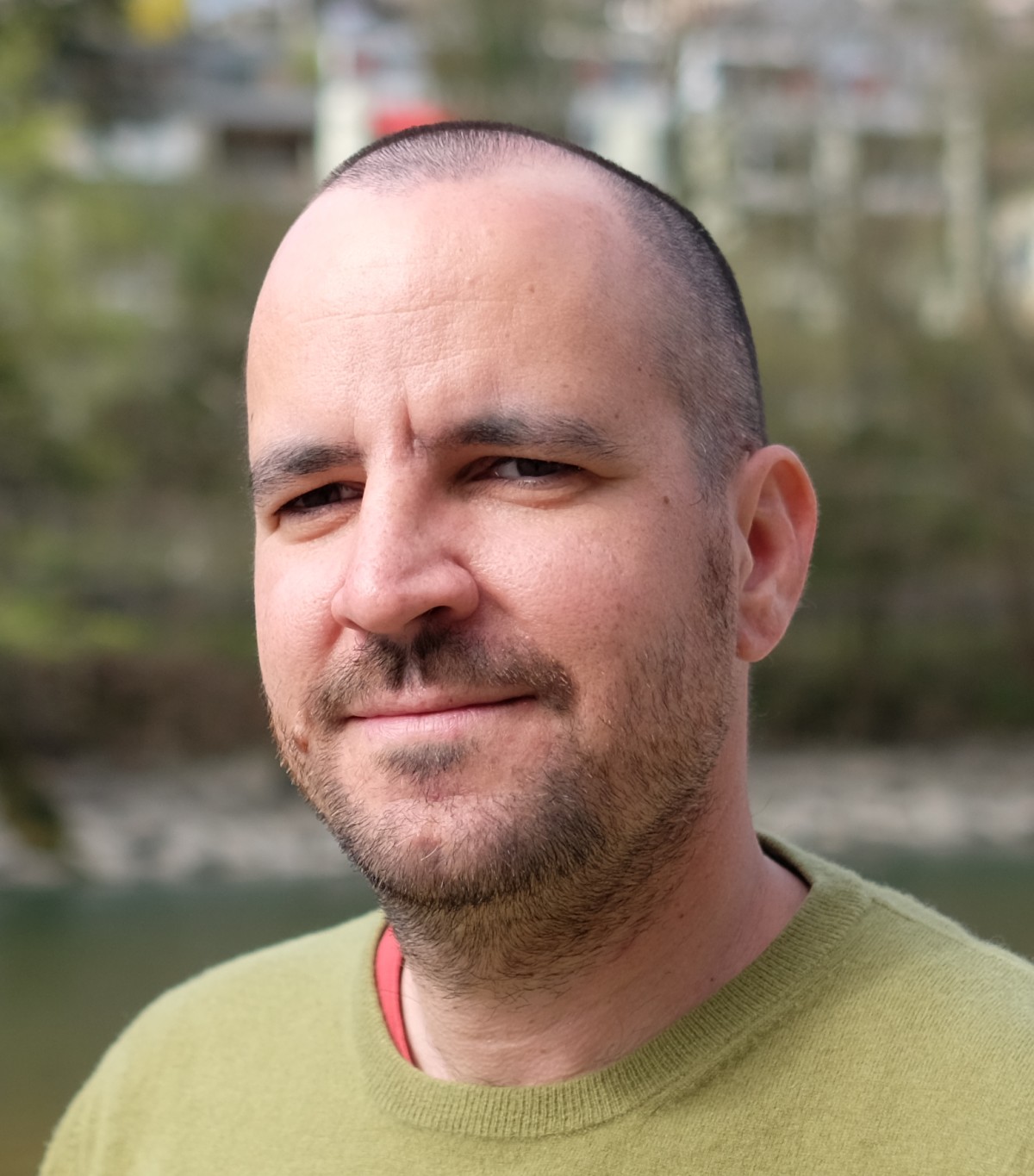
Dr. Lukas Roth
Lecturer at the Department of Environmental Systems Science
- Work phone +41 44 632 30 09
- Work phone +41 52 354 92 88 Alternate(Alt.)
- phone +41 44 632 38 79 Secretariat(Sec.)
- call_made0000-0003-1435-9535
- contactsV-Card (vcf, 1kb)
Additional information
Research area
Publications:
General:
My research focuses on high-throughput field phenotyping. While I mainly used drone-based platforms in the past years, I am now also including data collected with stationary platforms. I have developed a toolbox of low-level traits for wheat and soybean (e.g., LAI estimations). I further process those traits to intermediate traits of three types—timings, quantities and dose-response curve traits, to finally predict a target trait such as yield.
Plant breeding: Why and how to improve it?
Sustainable and local production of crops requires varieties that are highly adapted to local environmental conditions. Resilience to climate change has become a major issue in crop cultivation. Pressure on breeders is high to provide better adapted cultivars. New high-throughput field phenotyping (HTFP) technologies can contribute to the needed increase in efficiency in breeding. A main strategy to bring HTFP into breeding is the physiological breeding approach. In physiological breeding, one does not directly select for a target trait (e.g., yield or protein content) but defines physiologically related but easier-to-capture intermediate traits (e.g., early vigor).
Drones versus FIP: Highly mobile versus highly precise phenotyping platforms
Collecting HTFP data required platforms carrying sensors, e.g. consumer-grade RGB cameras. While I mainly focused on drone-based platforms in the past year, I am now also including data collected with the Field Phenotyping Platform (FIP) at ETH (https://kp.ethz.ch/infrastructure/FIP.html), unraveling the trade-of between mobility and precision.
Low-level traits: The foundation of automated phenotyping
HTFP requires extracting features—the so called low-level traits—from geospatial products, e.g. plant height, canopy cover, and other measures that describe phenotypes. I have developed a toolbox of these low-level traits for wheat and soybean, two crops among the most important crops for food respectively fodder production worldwide. I am constantly extending this toolbox, thereby switching the focus to machine learning methods.
Dynamics traits: Facilitator for physiological breeding approaches
Monitoring breeding experiments with high-throughput (up to 2–3 collection campaigns per week) results in dense time series of low-level traits. Extracting the dynamics of growth from such time series is regarded as essential step to model intermediate traits that are related to target traits such as yield. I was involved in the development of a theoretical framework to extract intermediate traits of three types—(1) timing of key stages, (2) quantities at defined time points or periods, and (3) dose-response curves. Currently, my main focus lies on analyzing the dependency of growth on temperature (driven by my employment as Post-doc for the SNF project ‘Phenoflow’). Again, I see the most promising approach in combining machine learning with conventional growth modeling.
Honours
Year | Distinction |
---|---|
2021 | ETH medal for outstanding doctoral theses |
Course Catalogue
Spring Semester 2025
Number | Unit |
---|---|
751-3500-00L | Plant Breeding |